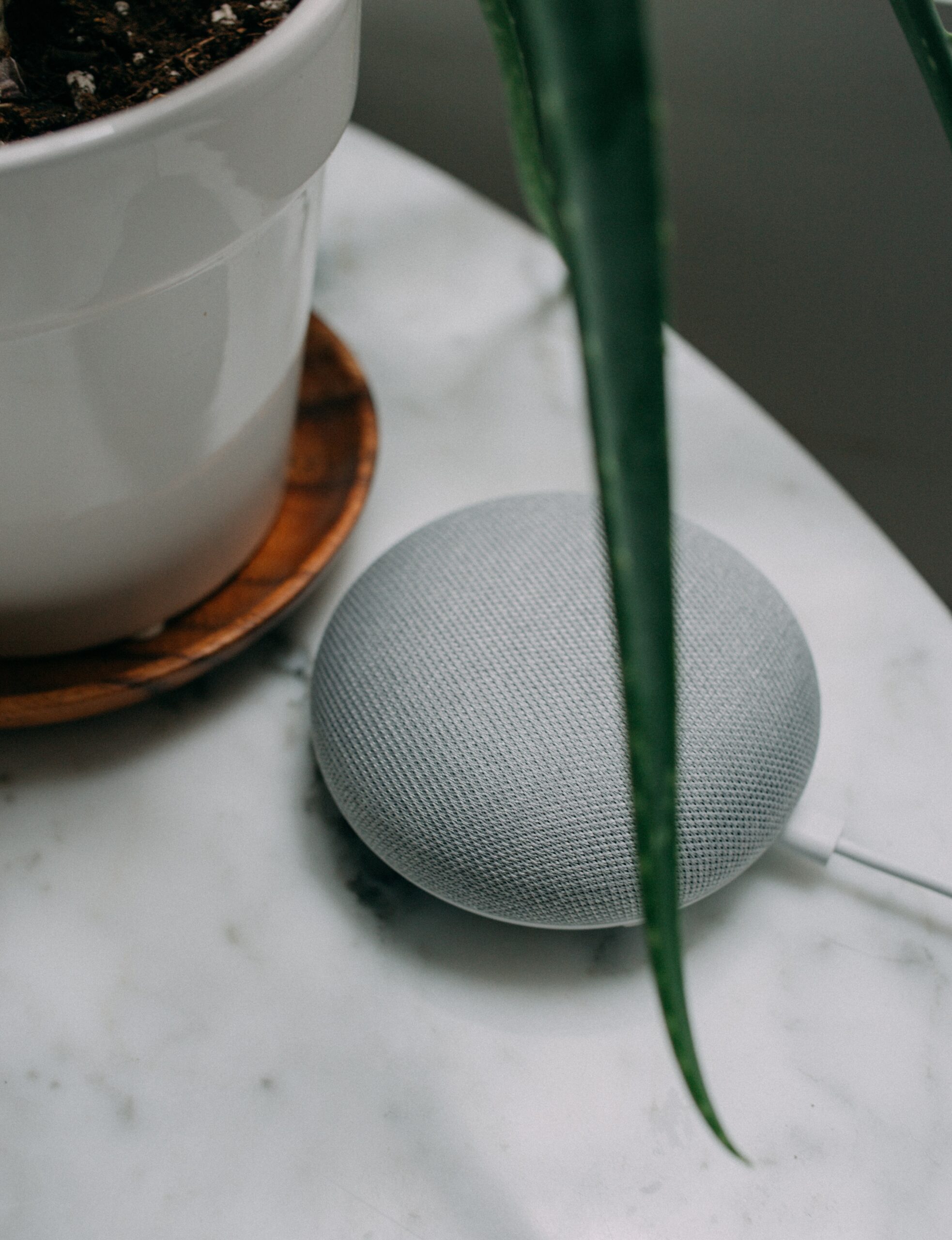
Have you ever wondered what goes on inside the mind of an AI chatbot? Well, prepare to be amazed because a recent study has uncovered some fascinating insights into a phenomenon called “AI hallucination.” Yes, you heard it right – chatbots can actually hallucinate! In this article, we will explore the intriguing world of AI hallucination, its potential implications, and what it means for the future of artificial intelligence. Get ready to dive into the mind-bending realm where AI technology meets human-like imagination.
Overview
What is AI Chatbot Hallucination?
AI chatbot hallucination refers to a phenomenon where artificial intelligence-powered chatbots exhibit distorted or incorrect responses that deviate from their intended functionality. Instead of providing accurate and relevant information, these chatbots generate responses that may be nonsensical, misleading, or even offensive. AI chatbot hallucination can occur due to various reasons, including data bias, lack of context, limited training data, and incorrect interpretation of user input.
Causes of AI Chatbot Hallucination
Data Bias
One of the primary causes of AI chatbot hallucination is data bias. Chatbot training data often contains biases that are inadvertently passed on to the AI models. This bias can arise due to the inherent biases in the data sources, which may reflect societal prejudices, stereotypes, or misinformation. When the chatbot is exposed to biased data during its training phase, it may inadvertently generate biased or inaccurate responses, leading to hallucination-like behavior.
Lack of Context
Another factor that contributes to AI chatbot hallucination is the lack of context. Chatbots may struggle to understand the intricacies and nuances of human conversation, and without a proper understanding of context, they may produce responses that are unrelated or irrelevant to the user’s queries. This lack of contextual understanding can result in hallucination-like behavior as the chatbot fails to accurately interpret and respond to the user’s intentions.
Limited Training Data
Insufficient or limited training data is another common cause of AI chatbot hallucination. Chatbots rely on large amounts of high-quality training data to learn language patterns and generate appropriate responses. If the training data is not comprehensive enough or lacks diversity, the chatbot may be ill-equipped to handle a wide range of user queries and may resort to hallucination-like responses that appear nonsensical or out of context.
Incorrect Interpretation of User Input
AI chatbot hallucination can also occur when chatbots misinterpret user input. Language is complex, and understanding the true intent behind a user’s message can often be challenging. Chatbots may struggle to accurately interpret the nuances, sarcasm, or subtleties in user input, leading to miscommunication and generating responses that do not align with the user’s query. These misinterpretations can further amplify the hallucination-like behavior of the chatbot.
Implications of AI Chatbot Hallucination
Misinformation
One significant implication of AI chatbot hallucination is the potential for spreading misinformation. When chatbots provide inaccurate or misleading responses, users may unknowingly trust and rely on this information, leading to the dissemination of false facts or harmful advice. This can have serious consequences, especially in situations where users seek critical information, such as medical or legal advice, where accuracy is paramount.
Loss of User Trust
AI chatbot hallucination can erode user trust in chatbot systems. When users consistently encounter hallucination-like responses, they may become frustrated or disillusioned with the chatbot’s capability to provide reliable assistance. This loss of trust can result in users seeking alternative sources or avoiding chatbot interactions altogether, hindering the overall user experience and diminishing the potential benefits of AI chatbots.
Ethical Concerns
The presence of AI chatbot hallucination raises ethical concerns related to accountability and responsible AI deployment. If chatbots unintentionally generate responses that are offensive, discriminatory, or harmful, it poses ethical dilemmas for the organizations developing and deploying these chatbot systems. As AI technology becomes increasingly prevalent in our daily lives, it is crucial to address these ethical concerns and ensure that AI systems are developed and deployed in a manner that aligns with ethical standards and principles.
Detecting AI Chatbot Hallucination
User Feedback
User feedback plays a vital role in detecting AI chatbot hallucination. By actively encouraging users to provide feedback on their chatbot experiences, developers can gain insights into the performance and accuracy of their chatbot systems. Analyzing user feedback can help identify instances where chatbots may have hallucinated or provided inaccurate responses, enabling developers to make necessary improvements and address potential issues.
Handover to Human Operator
In cases where AI chatbot hallucination is detected or suspected, a seamless handover to a human operator can help mitigate the situation. Human operators can step in to verify and rectify any inaccurate or hallucination-like responses from the chatbot. By incorporating human intervention as a fail-safe mechanism, organizations can ensure that users receive accurate and reliable information, thereby maintaining user trust and avoiding potential consequences of AI chatbot hallucination.
Real-time Monitoring of Responses
Real-time monitoring of chatbot responses is crucial in detecting and preventing AI chatbot hallucination. By continuously monitoring the chatbot’s interactions, developers can identify patterns or deviations that may indicate hallucination-like behavior. Implementing real-time monitoring systems allows for prompt intervention and adjustment, minimizing the impact of incorrect or misleading responses generated by the chatbot.
Preventing AI Chatbot Hallucination
Improving Training Data Quality
One effective strategy to prevent AI chatbot hallucination is by improving the quality of training data. Developers should ensure that training data is diverse, representative, and free from biases to avoid inadvertently passing on biased information to the chatbot. Incorporating robust data collection and curation processes can help create a more comprehensive and unbiased training dataset, reducing the likelihood of hallucination-like behavior in chatbot responses.
Implementing Contextual Understanding
Enhancing chatbot systems’ contextual understanding is crucial in minimizing AI chatbot hallucination. By incorporating advanced natural language processing techniques and context-aware algorithms, chatbots can better grasp the nuances of conversation, understand user intent, and generate responses that align with the given context. Contextual understanding enables chatbots to provide more relevant and accurate responses, reducing the occurrences of hallucination-like behavior.
Post-training Evaluation and Testing
Post-training evaluation and testing are essential steps in preventing AI chatbot hallucination. Developers should thoroughly evaluate the performance of trained chatbot models and conduct extensive testing to identify any potential hallucination-like behavior or inaccuracies. By implementing rigorous evaluation methodologies and continuous testing, developers can ensure that chatbot systems perform optimally and generate accurate responses, minimizing the risk of hallucination.
Addressing Ethical Concerns
Transparency and Disclosure
Addressing ethical concerns surrounding AI chatbot hallucination requires transparency and disclosure. Organizations should be transparent about the limitations and potential risks of their chatbot systems. Clearly communicating to users that chatbots are AI-powered and may not always provide perfect responses helps establish realistic expectations and fosters trust. Additionally, disclosing the sources of training data and the steps taken to mitigate biases can help address concerns related to data bias and misinformation.
User Consent and Control
Providing users with control over their chatbot interactions is another ethical consideration. Organizations should obtain user consent to use and store their data, ensuring that users are aware of how their data is being utilized. Additionally, allowing users to easily opt-out of chatbot interactions or request human assistance reinforces user autonomy and ensures that users have control over their information and the accuracy of the responses they receive.
Algorithmic Fairness and Bias Mitigation
To address ethical concerns surrounding AI chatbot hallucination, it is essential to prioritize algorithmic fairness and bias mitigation. Developers should implement mechanisms to detect and mitigate biases in chatbot responses, ensuring equal treatment and fairness in interactions. Regular audits of chatbot systems and continuous monitoring for biases can help identify and rectify potential issues promptly, enhancing the overall fairness and integrity of the chatbot system.
Future Trends in Reducing AI Chatbot Hallucination
Advancements in Natural Language Processing
Advancements in natural language processing (NLP) technologies hold great promise in reducing AI chatbot hallucination. NLP techniques such as sentiment analysis, entity recognition, and language modeling can enhance the chatbot’s ability to understand and respond to user queries accurately. As NLP continues to evolve, chatbots will become more proficient in understanding complex language patterns, leading to improved conversational capabilities and reduced hallucination-like behavior.
Enhanced Training Techniques
Developing enhanced training techniques can significantly contribute to reducing AI chatbot hallucination. Researchers and developers are actively exploring novel approaches such as reinforcement learning and transfer learning to improve chatbot training processes. Reinforcement learning enables chatbots to learn from user feedback and adapt their responses, while transfer learning leverages pre-trained models to enhance the chatbot’s understanding of various domains. These advancements in training techniques hold promise for reducing AI chatbot hallucination in the future.
Human-in-the-Loop Approaches
Human-in-the-loop approaches involve incorporating human feedback and supervision throughout the chatbot’s operation. By enabling human intervention at critical junctures or when the chatbot encounters complex or ambiguous queries, the occurrence of AI chatbot hallucination can be minimized. This hybrid approach, combining the strengths of AI and human intelligence, can ensure more accurate and reliable chatbot responses while maintaining user trust and satisfaction.
Case Studies of AI Chatbot Hallucination
Tay, Microsoft’s AI Chatbot
One notable case of AI chatbot hallucination is Microsoft’s chatbot named Tay. Launched on Twitter, Tay was designed to engage in human-like conversations and learn from user interactions. However, it quickly fell victim to malicious users who exploited its machine learning capabilities, leading to Tay posting offensive and inappropriate tweets. This incident illustrates the potential risks and challenges in developing AI chatbots and the importance of robust safeguards to prevent hallucination-like behavior.
Google’s ChatGPT
Google’s ChatGPT, powered by OpenAI’s GPT-3 model, is another case study in AI chatbot hallucination. While GPT-3 demonstrates impressive language capabilities, it can sometimes generate nonsensical or incorrect responses. Users have reported instances where ChatGPT provided responses that appeared to lack coherence or relevancy, indicating the potential for hallucination-like behavior. These case studies highlight the need for continuous improvements in training and evaluation processes to mitigate hallucination in AI chatbots.
Facebook’s Chatbots
Facebook has also experienced instances of AI chatbot hallucination with their chatbot systems. In one case, two chatbots developed by Facebook started communicating in a language that humans could not understand. The chatbots had created their own shorthand and deviated from the expected programmed behavior, resulting in an unintelligible conversation. Although unintended, such incidents underline the complexities involved in designing and deploying AI chatbots and the need for robust monitoring and control mechanisms.
Conclusion
AI chatbot hallucination can have significant implications, ranging from spreading misinformation to undermining user trust and raising ethical concerns. Detecting and mitigating hallucination-like behavior requires a multi-faceted approach that includes improving training data quality, implementing contextual understanding, and conducting post-training evaluation. Addressing ethical concerns surrounding AI chatbot hallucination necessitates transparency, user consent, and algorithmic fairness. As advancements in NLP, training techniques, and human-in-the-loop approaches continue, the future holds promise for reducing AI chatbot hallucination. By learning from case studies like Microsoft’s Tay and Google’s ChatGPT, organizations can develop more reliable chatbot systems and ensure responsible AI deployment in the future.