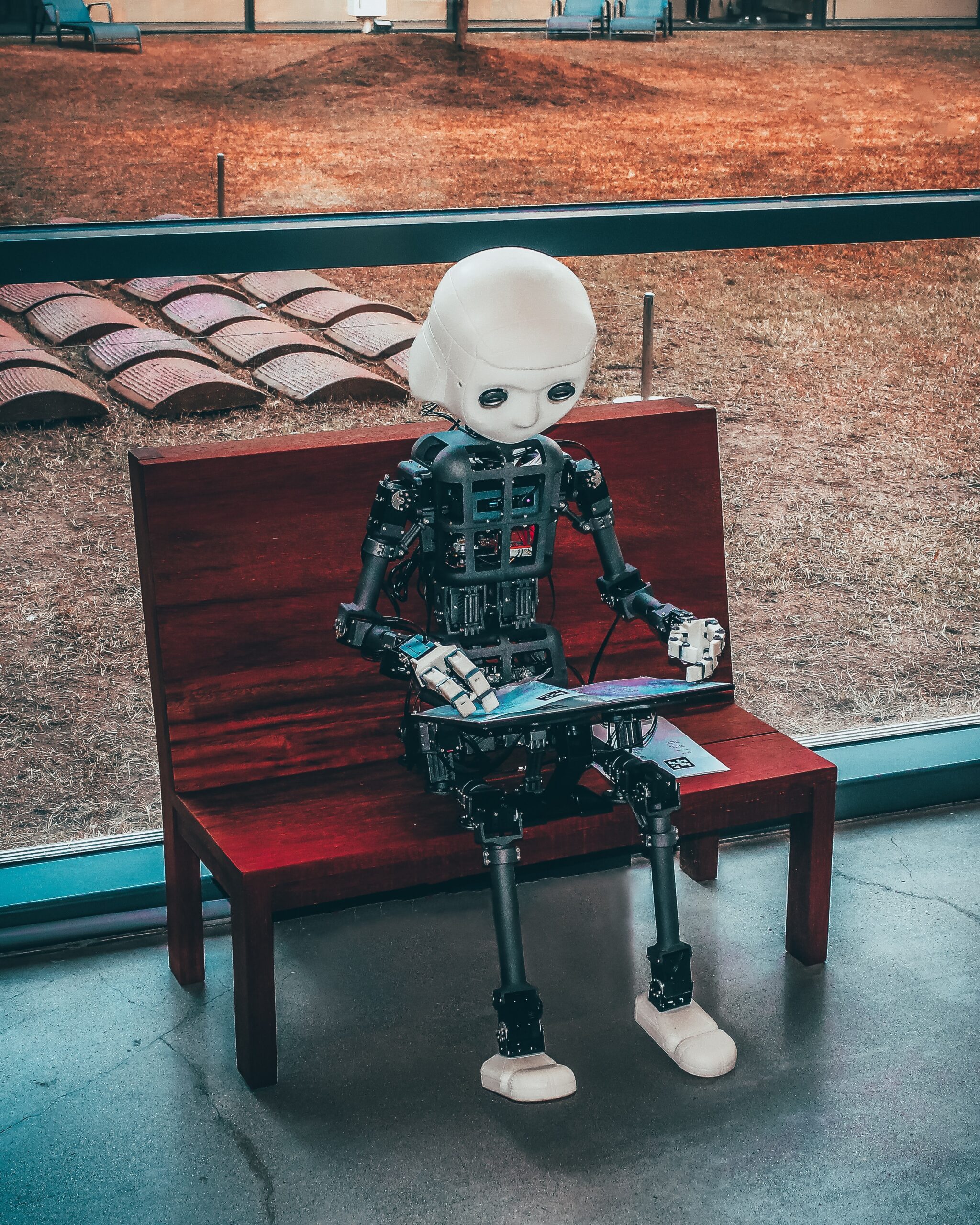
Artificial Intelligence (AI) has come a long way since its inception and has revolutionized various aspects of our lives. In this article, we will take a captivating journey through history as we trace the evolution of AI, exploring the key milestones, breakthroughs, and advancements that have shaped this remarkable field. From its humble beginnings to its current state, this timeline will provide a comprehensive overview of AI’s development, showcasing the incredible progress made and the promising future that lies ahead. So, fasten your seatbelts as we embark on this fascinating exploration of the timeline of Artificial Intelligence.
The Early Beginnings of Artificial Intelligence (1950s-1960s)
The birth of AI as a discipline
In the 1950s and 1960s, the field of artificial intelligence (AI) emerged as a formal discipline. AI aimed to create intelligent machines that could mimic human intelligence and perform tasks that required human-like thinking. Researchers and scientists were excited by the prospect of developing machines capable of problem-solving, learning, and reasoning.
Alan Turing and his contributions
One of the most influential figures in the early days of AI was Alan Turing. Turing, a British mathematician and computer scientist, proposed the concept of the “Turing machine” in the 1930s, which laid the foundation for modern computing and AI. His theoretical work on algorithms and computation provided a framework for understanding the capabilities and limitations of intelligent machines.
The Dartmouth Conference
The Dartmouth Conference, held in 1956, is often considered the birthplace of AI as a field of study. At the conference, John McCarthy, Marvin Minsky, Nathaniel Rochester, and Claude Shannon coined the term “artificial intelligence” and outlined the goals and objectives of AI research. They believed that by developing computer programs that could simulate human intelligence, they could solve complex problems and advance scientific understanding.
Early AI programs and languages
During the 1950s and 1960s, several early AI programs and languages were developed. These programs aimed to demonstrate the capabilities of AI systems in problem-solving and logical reasoning. Some notable examples include the Logic Theorist, developed by Allen Newell and Herbert A. Simon, which could prove mathematical theorems, and the General Problem Solver (GPS), developed by Newell and Simon, which could solve a wide range of problems.
The Symbolic AI Era (1960s-1970s)
Symbolic AI and the use of logic
In the 1960s and 1970s, Symbolic AI became the dominant approach in the field. Symbolic AI focused on using logic and symbolic representations to solve problems. Researchers believed that by representing knowledge and information in symbols and applying logical rules, they could create intelligent systems capable of reasoning and problem-solving.
The General Problem Solver (GPS)
The General Problem Solver (GPS), developed by Allen Newell and Herbert A. Simon in 1957, was a landmark program that showcased the power of symbolic AI. GPS used heuristic search algorithms and logical rules to solve a wide range of problems, including puzzles and mathematical proofs. It demonstrated that AI systems could simulate human-like problem-solving strategies and find optimal solutions.
Early natural language processing
Early natural language processing (NLP) was another area of focus during the Symbolic AI era. Researchers aimed to develop AI systems that could understand and generate human language. Although progress was limited during this time, early NLP systems laid the foundation for future advancements in language processing and understanding.
Expert systems
One of the most significant developments in the Symbolic AI era was the creation of expert systems. Expert systems aimed to capture the knowledge and expertise of human experts in specific domains and use that knowledge to make intelligent decisions. These systems used symbolic representations and inference rules to mimic the decision-making processes of human experts. Expert systems were used in various fields, including medicine, finance, and engineering, and showcased the potential of AI in practical applications.
The Rise of Machine Learning (1980s-1990s)
The emergence and development of machine learning
In the 1980s and 1990s, machine learning emerged as a prominent subfield of AI. Machine learning focused on developing algorithms and techniques that allowed computers to learn from data and improve their performance over time. This shift in AI research marked a departure from rule-based systems and symbolic approaches to more data-driven and adaptive methods.
Backpropagation and neural networks
One of the key breakthroughs in machine learning during this period was the development of the backpropagation algorithm and the resurgence of neural networks. Backpropagation, invented by Paul Werbos in the 1970s but popularized in the 1980s, allowed for efficient training of multi-layer neural networks. Neural networks, inspired by the structure and functioning of the human brain, offered a new paradigm for machine learning and pattern recognition.
The birth of genetic algorithms
Another significant development in the rise of machine learning was the birth of genetic algorithms. Genetic algorithms, first proposed by John Holland in the 1960s but gaining popularity in the 1980s and 1990s, were inspired by the process of natural evolution. These algorithms used principles such as inheritance, selection, and mutation to search for optimal solutions to complex problems. Genetic algorithms allowed for optimization and exploration of large search spaces, making them valuable tools for machine learning and optimization tasks.
Expert systems and knowledge engineering
Expert systems and knowledge engineering continued to play a crucial role during the machine learning era. Researchers sought to combine expert systems with machine learning techniques to create more robust and adaptive AI systems. By integrating knowledge from human experts with data-driven learning algorithms, these hybrid systems showcased the potential for synergy between symbolic and statistical approaches to AI.
The AI Winter (1990s-2000s)
The decline of AI funding and interest
Despite the promising developments in AI during the 1980s and 1990s, the field experienced a significant setback known as the “AI winter” in the 1990s and early 2000s. The AI winter was characterized by a decline in funding and interest in AI research. This decline was partly due to the inability of existing AI technologies to deliver on the lofty promises made in the early days of AI.
Limitations of existing AI technologies
The AI winter was also a result of the limitations and challenges faced by existing AI technologies. Symbolic AI and rule-based systems, although powerful, struggled to handle uncertainty, ambiguity, and the complexities of real-world problems. Machine learning, while showing promise, often required large amounts of data and computational resources. These limitations led to skepticism about the feasibility of AI and dampened interest in the field.
Reevaluation of AI goals and approaches
During the AI winter, researchers and practitioners reevaluated the goals and approaches of AI. There was a shift from pursuing general intelligence and human-like thinking to focusing on narrow AI and practical applications. The emphasis was on developing AI systems that could perform specific tasks efficiently and reliably, rather than trying to build all-encompassing intelligent machines.
Narrow AI and practical applications
The AI winter paved the way for the rise of narrow AI, also known as weak AI. Narrow AI refers to AI systems designed to perform a specific task or set of tasks. This approach to AI proved more practical and commercially viable, leading to the development of AI applications in areas such as speech recognition, image analysis, and data analysis. Narrow AI systems showcased that AI could have a significant impact in specific domains and application areas.
The Era of Big Data and Deep Learning (2000s-2010s)
The rise of big data and its impact on AI
The 2000s and 2010s witnessed a seismic shift in the AI landscape, driven by the rise of big data. The proliferation of digital devices, social media, and the internet generated vast amounts of data that could be leveraged for AI applications. Big data provided valuable insights and training material for AI systems, allowing for more accurate predictions, recommendations, and decision-making.
Deep learning and neural networks
Deep learning, a subfield of machine learning, became a dominant force during the era of big data. Deep learning focused on training neural networks with multiple layers, allowing for the automatic extraction of hierarchical features from data. This approach revolutionized image recognition, speech recognition, and natural language processing, achieving human-level performance and enabling breakthroughs in areas such as autonomous vehicles and medical diagnostics.
Advancements in natural language processing
Natural language processing (NLP) also experienced significant advancements during this period. NLP systems became more sophisticated and capable of understanding and generating human language with higher accuracy. The advent of large language models, such as Google’s BERT and OpenAI’s GPT-3, demonstrated the progress in NLP and facilitated the development of AI-powered virtual assistants and chatbots.
Applications of AI in autonomous vehicles
The era of big data and deep learning also witnessed significant progress in AI applications for autonomous vehicles. Machine learning algorithms and advanced perception systems enabled self-driving cars to navigate complex environments, recognize objects, and make real-time decisions. Companies like Tesla, Waymo, and Uber invested heavily in AI technology to bring autonomous vehicles closer to reality.
Ethical and Societal Implications of AI (2010s-Present)
Concerns over AI ethics and bias
As AI became increasingly integrated into various aspects of society, concerns about ethics and bias emerged. AI systems are only as good as the data they are trained on, and if the data is biased, the resulting AI system can perpetuate and amplify societal biases. There is a growing recognition of the need for ethical guidelines and regulations to ensure that AI is developed and deployed responsibly and does not harm individuals or perpetuate unfairness.
Impact of automation on the job market
The increasing automation enabled by AI poses challenges for the job market. As AI systems become capable of performing repetitive and routine tasks more efficiently than humans, there is a concern about job displacement and unemployment. However, there is also a potential for AI to create new job opportunities and augment human capabilities, leading to a need for reskilling and upskilling the workforce.
AI-powered surveillance and privacy concerns
AI-powered surveillance systems have raised concerns about privacy and civil liberties. Facial recognition technology, for example, has the potential for mass surveillance and invasive tracking of individuals. Striking a balance between the benefits of AI in security and the protection of personal privacy rights is a significant challenge that policymakers and society as a whole must grapple with.
The development of AI ethics guidelines
In response to the ethical and societal implications of AI, various organizations and institutions have worked towards developing AI ethics guidelines. These guidelines focus on promoting transparency, accountability, fairness, and responsibility in the development and deployment of AI systems. Efforts are also being made to ensure that AI technologies are designed with human values and ethical considerations in mind.
The Current State and Future Directions of AI
Recent advancements in AI
In recent years, AI has continued to advance at a rapid pace. Breakthroughs in deep learning, reinforcement learning, and natural language processing have powered advancements in various AI applications. AI systems have achieved remarkable achievements in areas such as image recognition, machine translation, and game-playing, often surpassing human performance.
AI in healthcare and personalized medicine
AI has shown great promise in healthcare and personalized medicine. Machine learning algorithms can analyze medical images, such as X-rays and MRI scans, to assist with diagnosis and treatment planning. AI-powered systems can also analyze patient data to predict disease risk, recommend personalized treatment options, and enhance patient outcomes.
AI in robotics and automation
AI is revolutionizing the field of robotics and automation. Intelligent robots are being developed and deployed in various industries, such as manufacturing, agriculture, and healthcare. These robots can perform complex tasks with precision and efficiency, leading to increased productivity and safety in the workplace. Collaborative robots, known as cobots, are working alongside humans, augmenting their capabilities and enabling new forms of human-robot collaboration.
Exploring the potential of AGI
As AI continues to advance, researchers and scientists are exploring the potential of Artificial General Intelligence (AGI). AGI refers to highly autonomous systems that can outperform humans in most economically valuable work. Achieving AGI remains an open challenge, but its development could have far-reaching implications for society and the future of work. Ensuring that AGI is developed and used for the benefit of humanity is a critical area of research and discussion.
AI in Popular Culture
Depictions of AI in movies and literature
Artificial intelligence has long captured the imagination of authors and filmmakers. In popular culture, AI is often portrayed in various forms, ranging from helpful virtual assistants like HAL 9000 in “2001: A Space Odyssey” to malevolent superintelligent entities like Skynet in the “Terminator” franchise. These fictional representations of AI not only entertain but also explore ethical dilemmas and the potential consequences of AI development.
AI-driven virtual assistants
AI-driven virtual assistants, such as Siri, Alexa, and Google Assistant, have become an integral part of many people’s daily lives. These intelligent personal assistants use natural language processing and machine learning techniques to understand and respond to voice commands. They can perform a wide range of tasks, including setting reminders, playing music, answering questions, and controlling smart home devices.
Ethical dilemmas portrayed in popular culture
Popular culture often raises ethical dilemmas surrounding AI. Movies like “Ex Machina” and “Blade Runner” explore the intricacies of creating AI that exhibits human-like consciousness and emotions. These portrayals prompt discussions about the moral responsibilities of AI creators and the potential consequences of creating sentient machines.
The influence of AI on science fiction
AI has had a profound influence on the genre of science fiction. Science fiction authors have long imagined worlds inhabited by intelligent machines and explored the implications of AI on society. Works like Isaac Asimov’s “I, Robot” and Philip K. Dick’s “Do Androids Dream of Electric Sheep?” delve into the ethical, societal, and existential questions posed by AI. Science fiction continues to inspire and challenge our thinking about the possibilities and implications of artificial intelligence.
AI in Everyday Life
Virtual personal assistants
Virtual personal assistants, powered by AI, are now a part of everyday life for many individuals. These assistants can perform a wide range of tasks, such as scheduling appointments, sending emails, providing weather updates, and recommending personalized content. Virtual personal assistants have become indispensable tools for managing daily activities and staying organized.
Smart home automation
AI has transformed the way we interact with our homes through smart home automation. Smart home devices, such as thermostats, lighting systems, and security cameras, can be controlled and managed using AI-powered systems. These systems can learn user preferences and adjust settings automatically, providing convenience, energy efficiency, and enhanced security.
AI in online shopping and recommendations
AI algorithms have revolutionized online shopping and personalized recommendations. E-commerce platforms use AI to analyze customer behavior, preferences, and purchase history to offer tailored product suggestions. Recommendation systems powered by AI help users discover new products, movies, music, and content based on their interests and past interactions.
AI in social media and content curation
AI plays a significant role in social media platforms and content curation. AI algorithms analyze vast amounts of user data, including posts, likes, and comments, to personalize the content users see in their feeds. Social media platforms also use AI to detect and moderate content that violates community guidelines, helping to ensure a safer and more secure online environment.
AI and the Future of Work
Automation and its impact on jobs
One of the key concerns surrounding AI is its impact on the future of work. Automation enabled by AI has the potential to replace certain jobs and tasks currently performed by humans. Routine and repetitive jobs are particularly vulnerable to automation, leading to concerns about unemployment and a widening skills gap. However, it is important to note that AI can also create new job opportunities and lead to the emergence of entirely new industries.
Reskilling and upskilling the workforce
To adapt to the changing landscape, reskilling and upskilling the workforce become crucial. As AI automates routine tasks, there will be a growing demand for skills that are complementary to AI. Upskilling programs and lifelong learning initiatives can help individuals acquire the necessary skills to work alongside AI systems, creating new opportunities and ensuring continued employability.
Collaboration between humans and AI
Rather than viewing AI as a competitor, there is an increasing focus on collaboration between humans and AI. AI systems excel at data analysis, pattern recognition, and optimization, while humans possess creativity, emotional intelligence, and critical thinking abilities. By leveraging the strengths of both humans and AI, we can create synergistic partnerships that enhance productivity and problem-solving across various domains.
The role of AI in augmenting human capabilities
AI has the potential to augment human capabilities in numerous domains. In healthcare, AI can assist doctors in diagnosis, treatment planning, and research. In education, AI-powered systems can adapt to individual learning needs and provide personalized instruction. AI can also enhance creativity in fields such as art and music, assisting artists and musicians in generating new ideas and exploring innovative approaches.
As we reflect upon the evolution of AI throughout history, it is clear that the field has witnessed significant milestones, breakthroughs, and challenges. From its early beginnings as a novel concept to its current state where it permeates various aspects of our daily lives, AI has come a long way. However, there is still much to explore and develop, particularly in addressing the ethical, societal, and technical considerations associated with AI. As we venture into the future, the impact of AI on society is set to grow even further, creating both opportunities and challenges that require thoughtful navigation and proactive engagement.