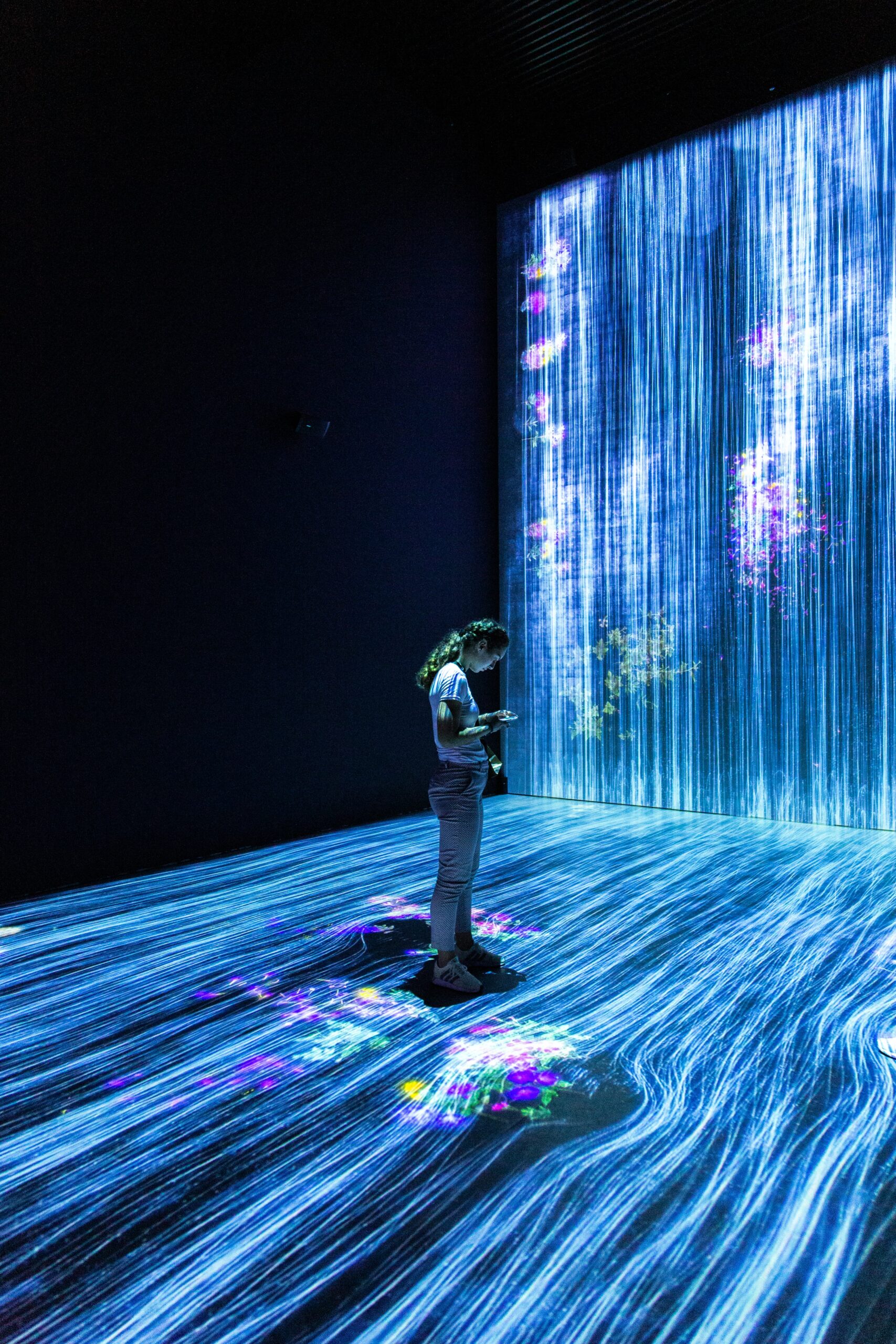
In this article, we will explore the exciting advancements and breakthroughs shaping the future of deep learning in neural network technologies. Delving into the latest trends and innovations, you will discover how these technologies are revolutionizing industries such as healthcare, finance, and transportation. With a friendly and accessible tone, we will dive into the fascinating world of deep learning and explore the potential it holds for transforming our lives. So get ready to embark on a captivating journey into the future of neural networks!
Trends in Deep Learning
Deep learning, a subset of machine learning, has seen tremendous growth and adoption in recent years. This surge in popularity can be attributed to the increasing access to vast amounts of data, powerful computing hardware, and advancements in algorithm development. As a result, deep learning has become a cornerstone technology in various industries, revolutionizing how we approach complex tasks and problems. Let’s delve into some of the key trends shaping the future of deep learning.
Increasing Adoption of Deep Learning
One of the most significant trends in deep learning is its widespread adoption across various sectors. From healthcare to finance, transportation to retail, organizations are recognizing the potential of deep learning to extract value from their data and gain a competitive edge. The ability of deep learning algorithms to automatically learn and extract meaningful insights from large datasets has opened up new possibilities for businesses, enabling them to make more informed decisions and uncover hidden patterns.
Expansion of Deep Learning in Different Industries
Deep learning is not limited to a specific industry; rather, it is making its presence felt in almost every sector. In healthcare, deep learning is being utilized for medical image analysis, disease diagnosis, drug discovery, and personalized medicine. Financial institutions leverage deep learning models for fraud detection, risk assessment, and algorithmic trading. Moreover, the automotive industry is harnessing the power of deep learning for self-driving cars and intelligent transportation systems. The widespread expansion of deep learning across industries reflects its potential to disrupt and transform traditional practices.
Advancements in Deep Learning Algorithms
The field of deep learning is constantly evolving with continuous advancements in algorithms. Researchers and practitioners are constantly experimenting with new architectures and techniques to improve the performance of deep learning models. Notable advancements include convolutional neural networks (CNNs) for image processing, recurrent neural networks (RNNs) for sequential data analysis, and transformers for natural language processing tasks. These innovations have pushed the boundaries of what deep learning can achieve and enabled more accurate, efficient, and versatile models.
Improved Hardware Capabilities for Deep Learning
Deep learning heavily relies on computational power to train complex models. To meet this demand, hardware advancements have played a crucial role. Graphics processing units (GPUs) have been widely adopted due to their parallel processing capabilities, enabling faster training and inference times. Additionally, dedicated tensor processing units (TPUs) have been developed specifically for deep learning tasks, further accelerating the training process. The availability of affordable, high-performance hardware has made deep learning accessible to a broader audience and fueled its growth.
Integration of Deep Learning with Other Technologies
Deep learning is often integrated with other emerging technologies, amplifying its impact and expanding its capabilities. For instance, the integration of deep learning with computer vision has led to significant advancements in areas such as object detection, image recognition, and video analysis. Similarly, the fusion of deep learning with natural language processing has facilitated the development of chatbots, virtual assistants, and sentiment analysis tools. The synergy between deep learning and these technologies has fostered a multidisciplinary approach to problem-solving and opened up new avenues for innovation.
Focus on Explainable and Interpretable AI
While deep learning models have achieved impressive results, they are often referred to as “black boxes” due to their lack of transparency and interpretability. Recognizing the need for explainable AI, researchers have been working on developing methods to interpret and understand the decisions made by deep learning models. This trend emphasizes the importance of not only achieving high accuracy but also providing insights into the reasoning behind the model’s predictions. Explainable AI ensures that deep learning models are not perceived as arbitrary solutions, but rather as trustworthy tools for decision-making in critical applications.
Innovations in Neural Network Technologies
As deep learning continues to evolve, new and innovative neural network technologies are being developed. These advancements are further enhancing the capabilities of deep learning models and expanding their applications. Let’s explore some of the notable innovations in neural network technologies.
Introduction of Capsule Networks
Capsule networks introduce a novel architectural concept that aims to address the limitations of traditional convolutional neural networks (CNNs). The idea behind capsule networks is to encapsulate information within capsules, which represent specific features of an input. These capsules enable better hierarchical representation learning, allowing the model to capture more robust and nuanced information. Capsule networks have shown promising results in tasks such as image recognition, with the potential for broader applications in the future.
Reinforcement Learning and Neural Networks
Deep reinforcement learning combines the power of deep neural networks with reinforcement learning algorithms. This approach enables the use of neural networks to learn to make decisions and take actions by interacting with an environment. Reinforcement learning has achieved remarkable success in challenging tasks, such as playing complex games like AlphaGo and mastering robotic control. The combination of reinforcement learning and deep neural networks holds immense potential for solving complex real-world problems that require decision-making and action-taking capabilities.
Generative Adversarial Networks (GANs)
Generative Adversarial Networks (GANs) have revolutionized the field of generative modeling. GANs consist of two neural networks, a generator and a discriminator, which compete against each other in a game-theoretic framework. The generator network learns to generate synthetic data that mimics a given dataset, while the discriminator network tries to distinguish between real and fake data samples. This adversarial training process leads to the refinement of both networks, resulting in the generation of highly realistic and diverse data samples. GANs have applications in various domains, including image and video synthesis, text generation, and data augmentation.
Graph Neural Networks
Graph neural networks (GNNs) have emerged as a powerful framework for analyzing and learning from graph-structured data. GNNs extend the traditional deep learning paradigm to handle complex relationships and dependencies present in graphs, such as social networks, molecular structures, and citation networks. By iteratively aggregating and updating information from neighboring nodes, GNNs can capture important graph-level patterns and make predictions based on the underlying connectivity. GNNs have shown notable success in tasks like node classification, link prediction, and graph generation.
Attention Mechanisms in Neural Networks
Attention mechanisms have gained significant attention in recent years for their ability to improve the performance of neural networks in various tasks. These mechanisms allow the model to dynamically focus on relevant parts of the input data, effectively allocating its computational resources for the most informative features. Attention mechanisms have been successfully applied in natural language processing for tasks such as machine translation, text summarization, and question-answering. Their integration into neural networks enhances their interpretability, efficiency, and overall performance.
Federated Learning
Federated learning addresses the challenge of training deep learning models on decentralized data. Rather than centralizing the data in a single location, federated learning enables training on data distributed across multiple devices or organizations while maintaining data privacy. This technology allows models to be learned collaboratively without the need to share raw data. Federated learning has implications in industries where data privacy is crucial, such as healthcare and finance, and has the potential to accelerate the development of robust and privacy-preserving deep learning applications.
Transfer Learning and Pretrained Models
Transfer learning has become a widely adopted technique in deep learning, enabling models to leverage knowledge gained from one task to perform well on another related task. Pretrained models, trained on large-scale datasets, provide a starting point for transferring knowledge and fine-tuning models for specific tasks. By utilizing learned representations from previous tasks, transfer learning significantly reduces the amount of data and computational resources required to achieve good performance. This approach has made deep learning more accessible and efficient, particularly in domains with limited data availability.
Autoencoders and Variational Autoencoders
Autoencoders are neural network architectures used for unsupervised learning and data compression. They consist of an encoder network that maps input data into a lower-dimensional latent space, and a decoder network that reconstructs the original data from the latent representation. Variational autoencoders (VAEs) take this concept further by introducing probabilistic encoders and decoders, which enable generative modeling and continuous latent space interpolation. Autoencoders and VAEs have applications in tasks such as image denoising, anomaly detection, and data generation.
Neuromorphic Computing
Neuromorphic computing aims to build computer systems that mimic the architecture and functionality of the human brain. By emulating the principles of neural networks with specialized hardware, neuromorphic computing offers potential advantages in terms of energy efficiency, parallelism, and cognitive capabilities. This technology has the potential to revolutionize artificial intelligence by enabling the development of highly efficient and brain-inspired computing systems. While still in its early stages, neuromorphic computing holds great promise for the future of deep learning and cognitive computing.
Brain-Computer Interfaces (BCIs)
Brain-Computer Interfaces (BCIs) establish a direct communication pathway between the brain and an external device, allowing individuals to control devices or interact with digital systems using their brain signals. Deep learning plays a crucial role in decoding and analyzing the complex patterns and signals generated by the brain. BCIs have applications in various domains, including healthcare, assistive technology, and gaming. The integration of deep learning with BCIs has the potential to enhance human-computer interaction and empower individuals with new ways of communication and control.
As the future of deep learning unfolds, these trends and innovations in neural network technologies will pave the way for groundbreaking advancements across industries. The increasing adoption of deep learning, coupled with the continuous development of algorithms and hardware capabilities, will further propel this field forward, enabling us to solve more complex problems, unlock new insights, and transform the way we live and work. With the ongoing focus on explainable and interpretable AI, deep learning models will continue to become more transparent, reliable, and trusted, ensuring that they serve as effective tools in enhancing decision-making and enabling us to harness the full potential of artificial intelligence.