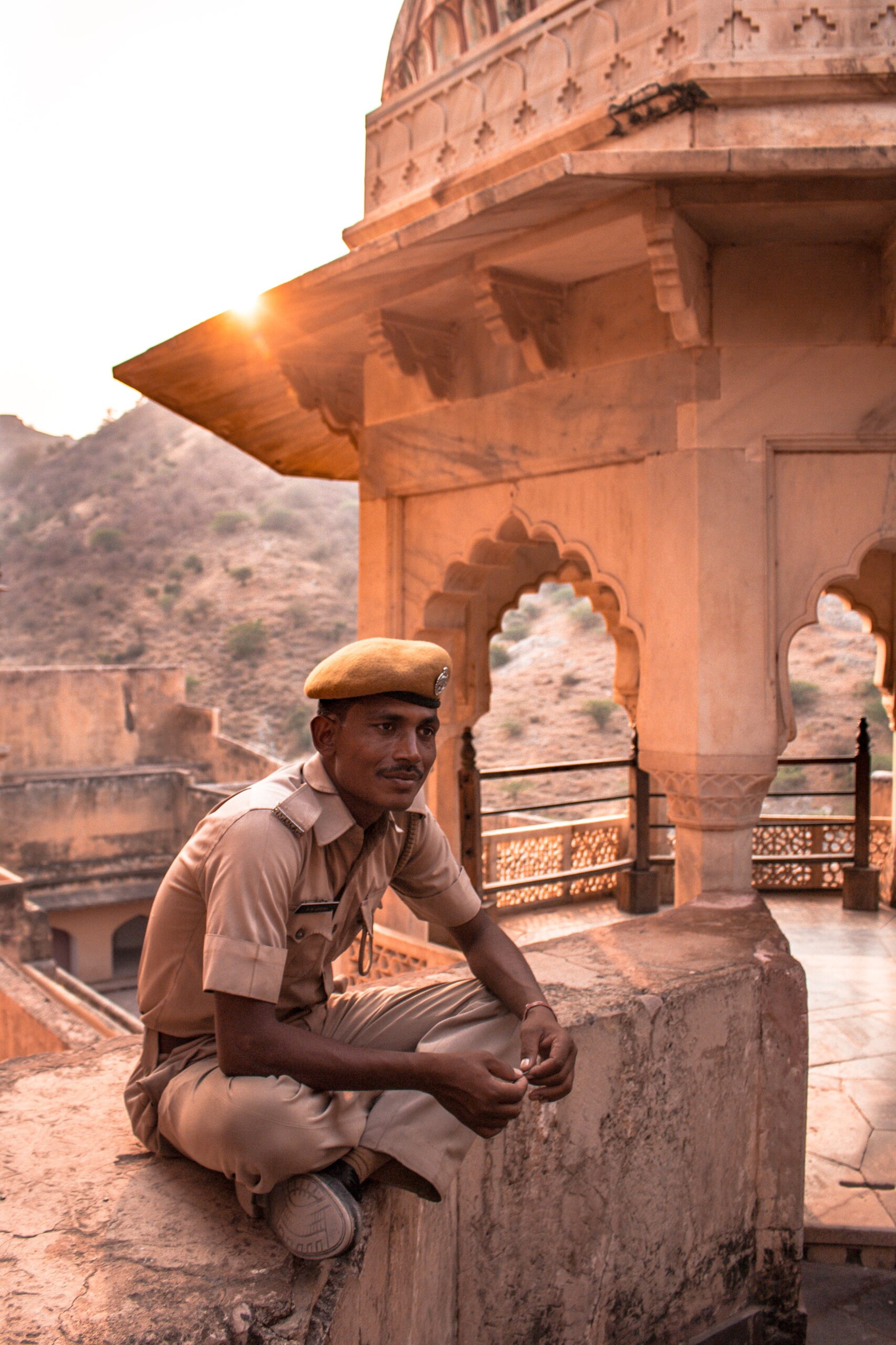
In today’s rapidly changing digital landscape, the integration of machine learning in risk management has become a pivotal tool in enhancing financial security and fraud detection. With the increasing complexity of financial transactions and the constant evolution of fraudulent activities, traditional risk management approaches alone are no longer sufficient. This article explores the powerful capabilities of machine learning and how it empowers organizations to efficiently identify and mitigate potential risks, while effectively safeguarding their financial assets.
Introduction to machine learning in risk management
Machine learning refers to the branch of artificial intelligence that enables computer systems to learn and improve from experience without being explicitly programmed. In the context of risk management, machine learning algorithms are utilized to analyze vast amounts of data and make predictions or decisions based on patterns, correlations, and historical data.
The importance of machine learning in risk management cannot be overstated. Traditional risk management techniques and models often fall short in accurately predicting and preventing emerging risks and fraudulent activities. Machine learning offers a more sophisticated and flexible approach that can adapt and evolve as new risks emerge.
Applications of machine learning in risk management
Fraud detection
One of the key applications of machine learning in risk management is fraud detection. Traditional methods of fraud detection are often rule-based and rely on pre-defined thresholds or criteria, which can be easily bypassed by sophisticated fraudsters. Machine learning algorithms, on the other hand, are capable of analyzing large volumes of data in real-time and identifying patterns and anomalies that may indicate fraudulent activities.
Credit risk assessment
Machine learning has revolutionized credit risk assessment by providing more accurate and timely predictions of default probability. By analyzing a wide range of variables, including credit scores, financial statements, and transaction histories, machine learning algorithms can assess the creditworthiness of applicants and assign appropriate risk ratings.
Anti-money laundering (AML)
Machine learning plays a crucial role in anti-money laundering efforts by helping financial institutions identify and report suspicious transactions and entities. By monitoring customer behavior patterns and creating risk profiles, machine learning algorithms can detect unusual or suspicious activities and flag them for further investigation.
Transaction monitoring
Machine learning enables real-time monitoring of transactions to identify and prevent fraudulent activities. By analyzing large volumes of data and detecting fraud patterns, machine learning algorithms can accurately identify suspicious transactions and reduce false positives.
Cybersecurity
Machine learning is increasingly being used in cybersecurity to identify and prevent cyber threats. By analyzing network traffic and identifying anomalies, machine learning algorithms can detect and respond to potential attacks in real-time. Additionally, machine learning can be used for predictive analysis of vulnerabilities, allowing organizations to proactively address potential security risks.
Enhancing financial security through machine learning
By leveraging machine learning in risk management, financial institutions can enhance their financial security in several ways.
Identifying and preventing fraudulent activities
Machine learning algorithms can analyze vast amounts of data, including transaction records, customer information, and external data sources, to identify fraudulent activities. By detecting patterns and anomalies that may indicate fraud, machine learning models can help organizations take proactive measures to prevent financial losses.
Real-time monitoring and alerts
Machine learning algorithms can monitor transactions and activities in real-time, allowing organizations to respond promptly to suspicious activities. By setting up automated alerts and notifications, organizations can ensure that potential risks are identified and addressed immediately.
Improved accuracy in risk assessment
Machine learning can significantly improve the accuracy of risk assessment models by considering a wide range of variables and historical data. By analyzing patterns and correlations in data, machine learning algorithms can provide more accurate predictions of default probability, creditworthiness, and other risk factors.
Fraud detection using machine learning
Machine learning is particularly effective in detecting fraud, as it can leverage historical data to identify patterns and anomalies that may indicate fraudulent activities.
Training models with historical data
To build effective fraud detection models, machine learning algorithms are trained using historical data that includes both fraudulent and legitimate transactions. By analyzing the features and characteristics of both types of transactions, the algorithm can learn to distinguish between them and identify potential fraud.
Identifying patterns and anomalies
Machine learning algorithms use statistical techniques to identify patterns and anomalies in transaction data. By analyzing various variables, such as transaction amount, location, and customer behavior, machine learning models can identify unusual or suspicious activities that may be indicative of fraud.
Unsupervised and supervised learning algorithms
Machine learning algorithms for fraud detection can be categorized into unsupervised and supervised learning algorithms. Unsupervised learning algorithms analyze data without predefined labels, allowing them to capture unexpected patterns. Supervised learning algorithms, on the other hand, are trained using labeled data, which enables them to make predictions based on past observations.
Credit risk assessment with machine learning
Machine learning has significantly improved credit risk assessment by providing more accurate predictions of default probability and creditworthiness.
Assessing creditworthiness of applicants
Machine learning algorithms can analyze a wide range of variables, including credit scores, financial statements, and transaction histories, to assess the creditworthiness of loan applicants. By considering multiple factors and analyzing patterns in historical data, machine learning models can assign appropriate risk ratings to applicants.
Evaluating credit scores and histories
Machine learning algorithms can analyze credit scores and credit histories to evaluate the creditworthiness of individuals or organizations. By considering various factors, such as payment history, outstanding debts, and credit utilization, machine learning models can provide a more comprehensive assessment of credit risk.
Predicting default probability
Machine learning algorithms can also predict the probability of default based on historical data and various risk factors. By analyzing patterns and correlations in data, machine learning models can provide more accurate predictions, allowing organizations to make informed decisions about lending or credit decisions.
AML detection using machine learning
Machine learning is instrumental in detecting and preventing money laundering activities by monitoring transactions and customer behavior.
Identifying suspicious transactions and entities
Machine learning algorithms can analyze transaction data to identify suspicious transactions and entities. By analyzing various variables, such as transaction amount, frequency, and destination, machine learning models can detect unusual patterns that may be indicative of money laundering activities.
Monitoring customer behavior patterns
Machine learning algorithms can create risk profiles for customers based on their transaction history, behavior patterns, and external data sources. By monitoring these risk profiles, financial institutions can identify deviations from normal behavior and flag them for further investigation.
Creating risk profiles
Machine learning algorithms can create risk profiles for both individuals and entities by analyzing various data sources, such as transaction records, customer information, and external databases. These risk profiles can help financial institutions identify and assess potential money laundering risks, enabling them to take appropriate measures to mitigate these risks.
Transaction monitoring with machine learning
Machine learning enables real-time monitoring of transactions to identify and prevent fraudulent activities.
Identifying suspicious transactions in real-time
Machine learning algorithms can analyze transaction data in real-time and identify suspicious activities as they occur. By considering various variables, such as transaction amount, location, and customer behavior, machine learning models can quickly detect and flag potentially fraudulent transactions.
Analyzing large volumes of data for fraud patterns
Machine learning algorithms are capable of analyzing large volumes of transaction data to identify patterns and correlations that may indicate fraud. By leveraging advanced data analysis techniques, such as data mining and pattern recognition, machine learning models can detect complex fraud patterns that may be missed by traditional methods.
Reducing false positives
One of the major challenges in transaction monitoring is the high rate of false positives, where legitimate transactions are mistakenly flagged as suspicious. Machine learning algorithms can help address this issue by continuously learning from the data and improving the accuracy of fraud detection models. By reducing false positives, financial institutions can minimize disruption to legitimate transactions and improve overall operational efficiency.
Enhancing cybersecurity through machine learning
Machine learning is increasingly being used in cybersecurity to identify and prevent cyber threats.
Identifying and preventing cyber threats
Machine learning algorithms can analyze network traffic and detect patterns and anomalies that may indicate potential cyber threats. By continuously monitoring network activity and comparing it to historical data, machine learning models can identify suspicious activities and take proactive measures to prevent cyber attacks.
Anomaly detection in network traffic
Machine learning algorithms can detect anomalies in network traffic, such as unusual data flows or access patterns, which may be indicative of a cyber attack. By analyzing large volumes of network data and comparing it to normal behavior, machine learning models can identify deviations and trigger alerts for further investigation.
Predictive analysis of vulnerabilities
Machine learning can be used to predict potential vulnerabilities in cybersecurity systems by analyzing historical data and identifying patterns that may be indicative of future attacks. By proactively addressing these vulnerabilities, organizations can enhance their cybersecurity defenses and reduce the risk of successful cyber attacks.
Challenges and limitations of using machine learning in risk management
While machine learning offers significant benefits in risk management, there are also challenges and limitations that need to be considered.
Data quality and data sources
Machine learning models heavily rely on high-quality data for training and prediction. Obtaining reliable and accurate data can be a challenge, especially when dealing with large volumes of transactional and customer data. Additionally, ensuring the integrity and security of data sources is crucial to prevent unauthorized access or manipulation of data.
Interpretability of machine learning models
Machine learning models often operate as “black boxes,” making it difficult to interpret their decisions or predictions. This lack of interpretability can make it challenging to understand the rationale behind certain risk assessments or fraud detection outcomes. Ensuring transparency and explainability in machine learning models is crucial to gain stakeholders’ trust and confidence in their decisions.
Algorithmic biases and fairness
Machine learning models can be affected by biases that are present in the training data. Biases can result in unfair or discriminatory outcomes, such as biased credit decisions or unfair treatment of certain customer segments. Addressing and mitigating algorithmic biases is essential to ensure fairness and ethical use of machine learning in risk management.
Best practices for implementing machine learning in risk management
To ensure successful implementation of machine learning in risk management, organizations should follow best practices throughout the process.
Data preprocessing and feature engineering
Data preprocessing and feature engineering are critical steps in preparing data for machine learning models. This involves cleaning and formatting data, handling missing values, and transforming variables into appropriate formats. Additionally, feature engineering aims to identify and create relevant features that can improve the performance of machine learning models.
Model selection and validation
Selecting the appropriate machine learning model for a specific risk management task is essential. Different models have different strengths and weaknesses, and their suitability depends on the nature of the problem and the available data. It is also important to validate the selected model using appropriate evaluation metrics and techniques to ensure its effectiveness and reliability.
Continuous monitoring and updating
Machine learning models should be continuously monitored and updated to ensure their performance remains consistent and accurate over time. This involves monitoring model outputs, evaluating model performance, and updating the models as new data becomes available. Regular model maintenance and improvement are crucial to adapt to changing risk landscapes and emerging threats.
Implementing machine learning in risk management requires careful planning, consideration of data quality and sources, and adherence to ethical principles and fairness. By leveraging machine learning techniques, organizations can enhance their financial security, improve fraud detection capabilities, and make more accurate and informed risk assessments.