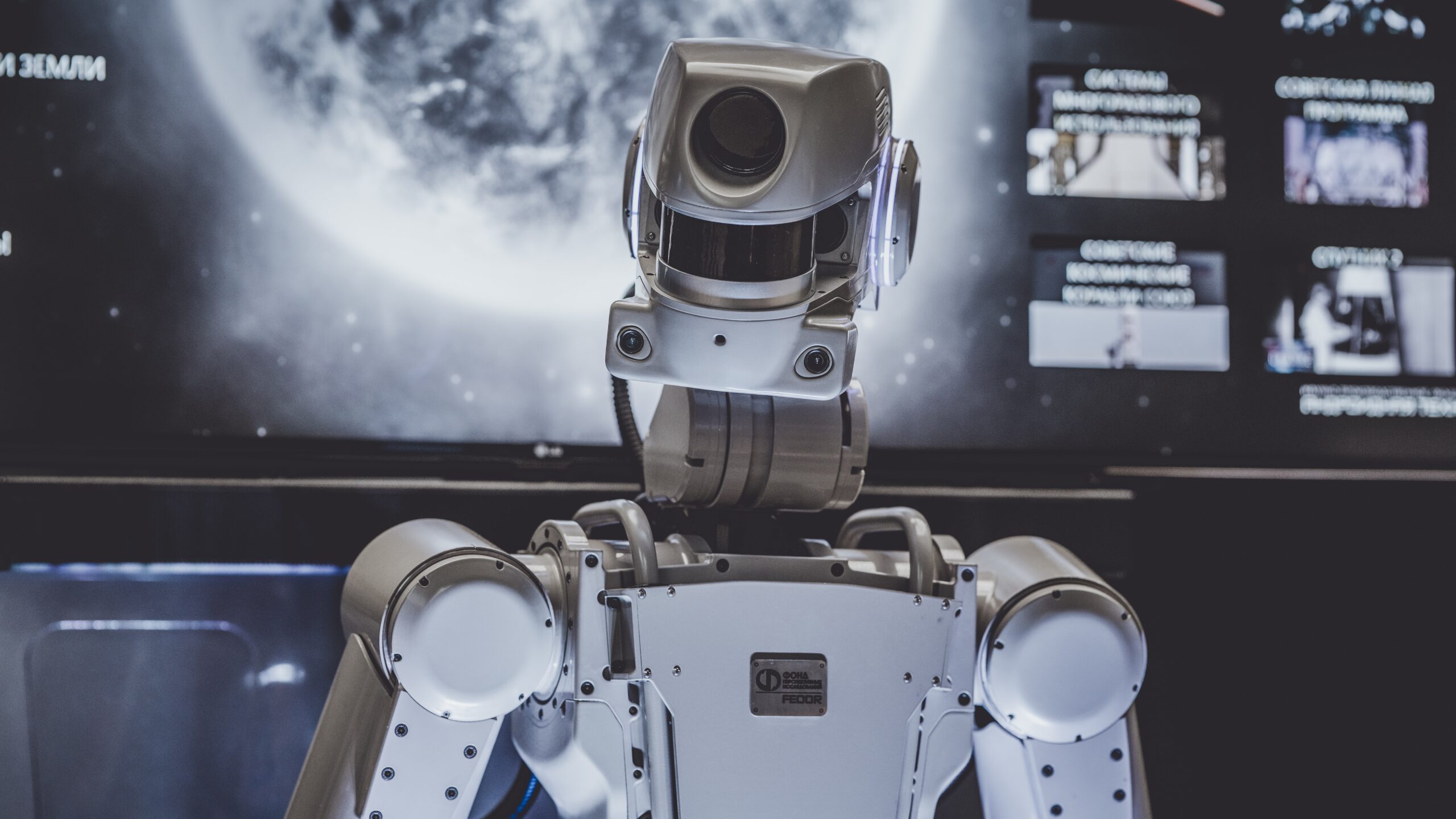
Imagine a world where driving is a thing of the past, where cars navigate the roads effortlessly and safely without any human intervention. This may seem like something out of a science fiction movie, but thanks to the remarkable advancements in deep learning, this futuristic vision is becoming a reality. Deep learning, a subset of artificial intelligence (AI), is a technology that is revolutionizing the future of transportation. By allowing vehicles to learn from vast amounts of data and make decisions based on their own experiences, deep learning is paving the way for fully autonomous vehicles that promise to transform the way we travel.
Understanding Deep Learning
Deep learning is a subfield of machine learning that focuses on training artificial intelligence (AI) systems to learn from data and make intelligent decisions. It involves training neural networks, which are computational models inspired by the human brain, to recognize patterns, process complex information, and make accurate predictions or decisions. With deep learning, AI systems can automatically learn and improve from experience without being explicitly programmed, enabling them to perform advanced tasks and solve complex problems.
Definition of Deep Learning
Deep learning is a subset of machine learning that utilizes neural networks with multiple layers to extract and learn complex representations from large amounts of data. These neural networks are often referred to as deep neural networks due to their multiple hidden layers. The depth of these networks allows them to learn hierarchical representations, enabling them to perform tasks such as image recognition, speech synthesis, natural language processing, and much more.
How Deep Learning Works
Deep learning algorithms are designed to automatically learn and detect meaningful patterns or features from data. The process starts with input data being fed into the neural network, which passes through multiple layers of interconnected neurons. Each neuron performs a simple calculation by applying a weight to the input and passing the result through an activation function. The outputs of the neurons in each layer are then used as inputs for the next layer, gradually transforming and combining the information until it reaches the final output layer.
During the training phase, deep learning models undergo an iterative process known as backpropagation. This process adjusts the weights and biases of the neural network based on the calculated error between the predicted output and the desired output. By minimizing this error through repeated training iterations, the neural network gradually learns to make more accurate predictions or decisions.
Autonomous Vehicles and Deep Learning
The integration of deep learning in autonomous vehicles has revolutionized the future of transportation. By harnessing the power of deep learning algorithms, autonomous vehicles can perceive their surroundings, make informed decisions, and navigate complex environments without human intervention. This technology opens up a world of possibilities for safer, more efficient, and more convenient transportation.
Integration of Deep Learning in Autonomous Vehicles
Deep learning plays a crucial role in enabling autonomous vehicles to perceive and understand their environment. Using various sensors such as cameras, lidar, radar, and GPS, the vehicle constantly collects a vast amount of data about its surroundings. Deep learning algorithms then analyze and interpret this data to identify objects, detect obstacles, recognize traffic signs, and understand the overall scene.
By integrating deep learning models into the autonomous vehicle’s software, it can accurately detect and classify objects in real-time. This allows the vehicle to recognize and respond to pedestrians, vehicles, cyclists, and other potential hazards on the road. Deep learning-based object detection algorithms have significantly improved the accuracy and reliability of perception systems in autonomous vehicles.
Advantages of Deep Learning in Autonomous Vehicles
Deep learning offers several advantages for autonomous vehicles:
-
Robust Perception: Deep learning algorithms excel at understanding complex visual and spatial information. This allows autonomous vehicles to accurately perceive and interpret their surroundings, even in challenging conditions such as low light, adverse weather, or occlusions.
-
Continuous Learning: Deep learning models can be continuously trained and improved as they gather more real-world data. This ensures that the autonomous vehicle’s perception system stays up to date with changing road conditions and new scenarios.
-
Real-Time Decision Making: Deep learning enables autonomous vehicles to make intelligent decisions in real-time based on their perception of the environment. This allows the vehicle to predict and respond to dynamic situations on the road, ensuring safety and efficient navigation.
-
Reduced Human Error: By removing the need for human intervention in the driving process, deep learning-powered autonomous vehicles can minimize the risk of human error, which is a leading cause of road accidents. These vehicles consistently follow traffic rules, maintain safe following distances, and make accurate judgments based on real-time data.
Technologies Used in Deep Learning for Autonomous Vehicles
Deep learning in autonomous vehicles relies on various technologies to achieve its goals. These technologies include:
Convolutional Neural Networks (CNN)
Convolutional Neural Networks (CNNs) are one of the fundamental deep learning architectures used for computer vision tasks in autonomous vehicles. CNNs excel at extracting and learning features from visual data, making them ideal for tasks such as object detection, image classification, and semantic segmentation.
CNNs utilize convolutional layers, pooling layers, and fully connected layers to process and analyze visual inputs. The convolutional layers extract local features by convolving input images with a set of learnable filters. Pooling layers downsample the extracted features to reduce computation while retaining important information. Finally, fully connected layers classify the extracted features, enabling the network to make predictions.
Recurrent Neural Networks (RNN)
Recurrent Neural Networks (RNNs) are a type of neural network architecture that excels at modeling sequential data. In autonomous vehicles, RNNs are often used for tasks such as natural language processing, speech recognition, and motion prediction.
RNNs have recurrent connections, allowing them to maintain memory of past inputs and incorporate context into their predictions. This makes them particularly suited for tasks that involve temporal dependencies, where the current state depends on past states. For example, an RNN can predict the future trajectory of a vehicle by considering its previous movements.
Generative Adversarial Networks (GAN)
Generative Adversarial Networks (GANs) are a type of deep learning architecture that consists of two neural networks: a generator and a discriminator. GANs are widely used in autonomous vehicles for tasks such as image synthesis, data augmentation, and anomaly detection.
The generator network is responsible for generating synthetic data samples, while the discriminator network aims to distinguish between real and synthetic samples. Both networks are trained simultaneously in a competitive manner. This adversarial training process leads to the generator network learning to produce increasingly realistic synthetic samples, while the discriminator network becomes better at distinguishing between real and synthetic data.
Long Short-Term Memory (LSTM)
Long Short-Term Memory (LSTM) is a variant of RNNs that overcomes the limitations of traditional RNNs in capturing long-term dependencies. LSTMs are widely used in autonomous vehicles for tasks such as trajectory prediction, speech recognition, and gesture recognition.
LSTMs have a more complex structure compared to traditional RNNs, incorporating memory cells and various gating mechanisms. These memory cells allow LSTMs to selectively remember or forget information over long sequences, enabling them to capture long-term dependencies. This makes LSTMs particularly useful for tasks that involve long-term temporal dependencies, such as predicting the movement of other vehicles on the road.
Enhancing Perception with Deep Learning
Deep learning techniques greatly enhance the perception capabilities of autonomous vehicles. By leveraging deep learning algorithms, autonomous vehicles can accurately identify and understand objects in their surroundings, leading to safer and more reliable autonomous driving.
Object Recognition and Detection
Object recognition and detection are essential tasks for autonomous vehicles. Deep learning algorithms enable vehicles to identify and classify objects such as pedestrians, cyclists, vehicles, traffic signs, and traffic lights. With convolutional neural networks (CNNs), autonomous vehicles can accurately detect and track these objects in real-time, allowing them to make informed decisions and respond to their environment effectively.
Semantic Segmentation
Semantic segmentation refers to the process of assigning a semantic class label to each pixel in an image. Deep learning models, such as fully convolutional neural networks (FCNs), have revolutionized semantic segmentation tasks in autonomous vehicles. By accurately segmenting the scene, autonomous vehicles can understand the layout of the environment and identify regions such as roads, sidewalks, buildings, and vegetation.
Scene Understanding
Deep learning techniques also enable autonomous vehicles to achieve a higher level of scene understanding. By analyzing and interpreting sensor data using deep neural networks, autonomous vehicles can understand complex scenes and infer the intentions and behaviors of other objects on the road. This allows the vehicle to anticipate and respond to potential risks or hazards, ensuring safe navigation.
Improving Decision-Making with Deep Learning
Deep learning techniques not only enhance perception but also improve the decision-making capabilities of autonomous vehicles. By leveraging deep learning algorithms, autonomous vehicles can analyze complex data, predict future scenarios, and make informed decisions in real-time.
Behavioral Cloning
Behavioral cloning is a technique in which an autonomous vehicle learns to imitate human driving behavior by training on a large dataset of expert demonstrations. Deep learning models, such as CNNs, can be trained to replicate the driving behavior exhibited by human drivers, allowing the vehicle to navigate roads, follow traffic rules, and make appropriate decisions based on observed human behavior.
Motion Planning
Motion planning involves determining the optimal path and trajectory for an autonomous vehicle to reach its desired destination while avoiding obstacles and adhering to traffic laws. Deep learning algorithms can assist in motion planning by analyzing sensor data, predicting the behavior of other objects on the road, and generating safe and efficient trajectories. This enables the vehicle to navigate through complex environments and make real-time decisions based on its understanding of the scene.
Reinforcement Learning
Reinforcement learning is a type of machine learning in which an agent learns to interact with an environment by receiving feedback in the form of rewards or punishments. Deep reinforcement learning algorithms, such as deep Q-networks (DQNs) and proximal policy optimization (PPO), have been successfully applied in autonomous vehicles to learn complex decision-making policies.
By iteratively exploring the environment and learning from experience, deep reinforcement learning algorithms enable autonomous vehicles to learn optimal driving strategies. These algorithms can learn to navigate unfamiliar scenarios, respond to dynamic changes in the environment, and optimize driving behavior based on long-term rewards, such as energy efficiency or passenger comfort.
Deep Learning in Sensor Fusion
Sensor fusion is the process of combining data from multiple sensors to create a comprehensive and accurate representation of the environment. Deep learning techniques play a crucial role in sensor fusion for autonomous vehicles, enabling them to effectively integrate and interpret data from various sensors.
Fusing Data from Various Sensors
Autonomous vehicles rely on a combination of sensors, including cameras, lidar, radar, GPS, and inertial measurement units (IMUs), to gather information about their surroundings. Deep learning algorithms can fuse data from these sensors to create a holistic view of the environment. By leveraging techniques such as deep sensor fusion, autonomous vehicles can integrate information from multiple sensors and improve the accuracy and robustness of perception systems.
Sensor Calibration and Synchronization
Ensuring accurate sensor calibration and synchronization is critical for reliable perception and decision-making in autonomous vehicles. Deep learning algorithms can be used to automatically calibrate and synchronize sensor data, reducing the need for manual intervention. By continuously learning and adapting to sensor calibration and synchronization variations, deep learning models contribute to maintaining accurate and reliable perception systems.
Deep Learning for Mapping and Localization
Accurate mapping and localization are essential for autonomous vehicles to navigate and operate safely. Deep learning techniques are increasingly being employed to address the challenges associated with mapping and localization in autonomous vehicles.
Simultaneous Localization and Mapping (SLAM)
Simultaneous Localization and Mapping (SLAM) is a key technique for building and updating maps in real-time while simultaneously localizing the vehicle within the map. Deep learning can enhance SLAM algorithms by providing more accurate and robust perceptual inputs, enabling autonomous vehicles to build and update maps with higher precision and reliability.
Map Updating and Maintenance
Maps used by autonomous vehicles need to be updated and maintained to reflect changes in the environment. Deep learning algorithms can analyze sensor data, compare it with existing maps, and identify discrepancies or changes. By using deep learning techniques, autonomous vehicles can update and maintain their maps autonomously, ensuring that their navigation systems are up to date and accurate.
Challenges and Limitations of Deep Learning in Autonomous Vehicles
While deep learning has revolutionized autonomous vehicles, there are several challenges and limitations that need to be addressed:
Safety and Reliability Concerns
Ensuring the safety and reliability of deep learning algorithms in autonomous vehicles is of paramount importance. Deep learning models are highly dependent on the quality and diversity of the training data. Insufficient or biased training data can lead to performance issues and safety hazards. Validating and testing deep learning models in a wide range of scenarios and edge cases is crucial to ensure their reliability and robustness.
Interpreting Black Box Models
Deep learning models often operate as black boxes, making it challenging to interpret their decision-making processes. This lack of interpretability can be a significant concern for autonomous vehicles, as understanding why a particular decision was made is essential for safety and liability purposes. Efforts are being made to develop interpretable deep learning models and techniques to address this limitation.
Data Labeling and Annotation
Deep learning models require large amounts of labeled data for effective training. However, labeling and annotating data for autonomous driving can be a time-consuming and labor-intensive process. Developing efficient and accurate data labeling techniques, as well as creating high-quality labeled datasets, is crucial to support the continued advancement of deep learning in autonomous vehicles.
Regulatory and Ethical Considerations
As deep learning continues to advance in autonomous vehicles, regulatory and ethical considerations become increasingly important. Autonomous vehicles are subject to various laws and regulations that govern their operation on public roads. Establishing a legal framework that addresses the specific challenges and requirements of deep learning-powered autonomous vehicles is crucial to ensure safety, accountability, and public trust.
Ethical dilemmas also arise in the decision-making processes of autonomous vehicles. For example, in situations where a collision is unavoidable, the vehicle may need to make a decision that could result in harm to different individuals. Determining how autonomous vehicles should prioritize and make such decisions poses significant ethical challenges that must be carefully addressed.
Current and Future Applications of Deep Learning in Autonomous Vehicles
Deep learning has numerous current and future applications in autonomous vehicles, paving the way for a new era of transportation:
Ride-Sharing and Transportation as a Service
Deep learning-powered autonomous vehicles have the potential to revolutionize the ride-sharing industry. With accurate perception and decision-making capabilities, autonomous vehicles can provide safe and reliable transportation services. This can lead to increased efficiency, reduced traffic congestion, and improved accessibility for individuals who are unable to drive, such as the elderly or disabled.
Cargo and Delivery Services
Autonomous vehicles equipped with deep learning algorithms can optimize cargo and delivery services. These vehicles can autonomously load and unload goods, plan efficient routes, and adapt to changing traffic conditions. Deep learning-powered cargo and delivery vehicles offer the potential for cost savings, increased productivity, and reduced environmental impact.
Public Transportation Systems
Deep learning can play a significant role in enhancing public transportation systems. Autonomous buses and trains can utilize deep learning algorithms for precise localization, obstacle detection, and route optimization. Implementing deep learning-powered public transportation systems can lead to increased efficiency, reduced emissions, and improved passenger safety and comfort.
In conclusion, deep learning is revolutionizing the future of transportation by enabling autonomous vehicles to perceive their surroundings accurately, make intelligent decisions, and navigate complex environments. The integration of deep learning in autonomous vehicles unlocks numerous advantages, such as robust perception, continuous learning, real-time decision-making, and reduced human error. Deep learning techniques, including CNNs, RNNs, GANs, and LSTMs, enhance perception, improve decision-making, enable sensor fusion, and support mapping and localization in autonomous vehicles. However, challenges and limitations surrounding safety and reliability, interpretability, and data labeling must be addressed. Regulatory and ethical considerations are also vital as deep learning-powered autonomous vehicles become more prevalent. The current and future applications of deep learning in autonomous vehicles extend to ride-sharing, cargo and delivery services, and public transportation systems, revolutionizing transportation as we know it.