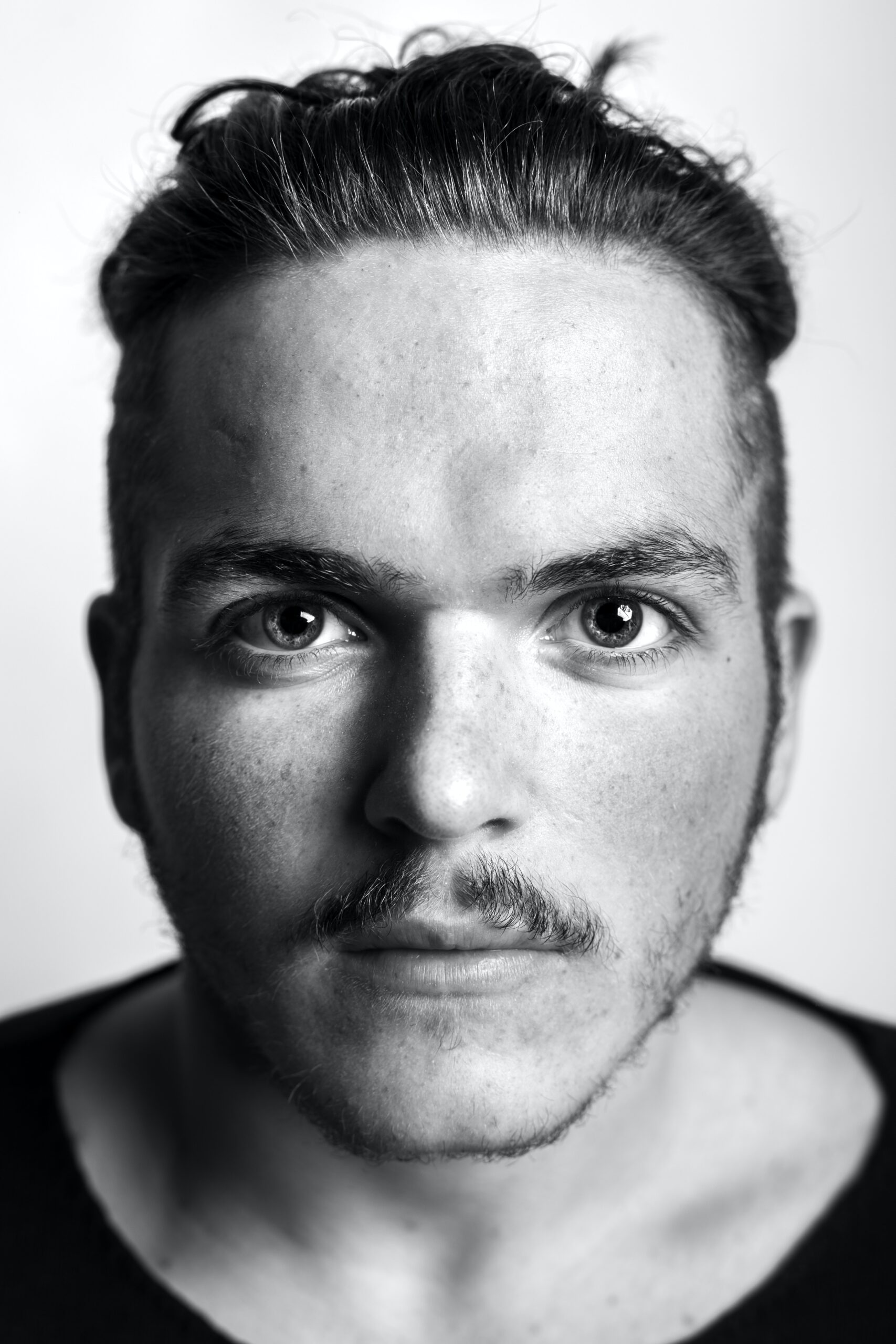
In the fast-paced world of finance, the use of artificial intelligence (AI) has become increasingly prevalent. However, this technological advancement brings with it a unique set of compliance and regulatory challenges. As financial institutions strive to leverage AI to enhance their operations and decision-making processes, it becomes crucial to ensure that ethical practices are upheld. This article delves into the key compliance and regulatory challenges faced by financial institutions in relation to AI, and explores the measures they can take to ensure ethical practices are maintained.
Overview of AI in Financial Institutions
Definition of AI in the context of financial institutions
Artificial intelligence (AI) refers to the development and deployment of intelligent machines and systems that can perform tasks and make decisions traditionally requiring human intelligence. In the context of financial institutions, AI technologies are utilized to analyze vast amounts of data, detect patterns and anomalies, develop predictive models, automate processes, and enhance decision-making capabilities. This includes applications such as fraud detection, credit scoring, trading algorithms, customer service bots, and risk management systems.
Emergence and development of AI in the financial sector
The use of AI in the financial sector has been rapidly growing in recent years. The emergence of big data, advancements in computing power, and the availability of sophisticated machine learning algorithms have paved the way for AI’s integration into various financial processes. Financial institutions are increasingly investing in AI technologies to gain a competitive edge, improve operational efficiency, enhance customer experience, and better manage risks. As AI continues to evolve, its role in transforming the financial landscape is only expected to expand further.
Benefits and potential risks associated with AI in financial institutions
The adoption of AI in financial institutions offers several potential benefits. Firstly, it enables institutions to process and analyze vast amounts of data in real-time, allowing for more accurate and timely decision-making. AI systems can detect complex patterns and anomalies that may go unnoticed by human analysts, resulting in more effective fraud detection and risk mitigation. Additionally, AI-powered chatbots and virtual assistants can provide personalized customer service, resolving queries and providing recommendations promptly.
However, there are also potential risks associated with AI in financial institutions. One major concern is the black-box nature of AI algorithms, which can make it difficult to understand and interpret their decision-making processes. This lack of transparency and explainability raises ethical concerns, particularly in cases where AI systems make crucial financial decisions. Biases in AI algorithms are another potential risk, as they can lead to discriminatory outcomes or perpetuate existing inequalities. Ensuring the ethical use of AI in financial institutions requires addressing these risks while harnessing the benefits it offers.
Regulatory Landscape for AI in Financial Institutions
Overview of existing regulations and guidelines
With the rapid advancement of AI in financial institutions, regulatory bodies and authorities are increasingly addressing the need for appropriate guidelines and regulations. Although comprehensive, globally accepted regulations for AI in finance are yet to be established, some jurisdictions have taken steps to outline specific guidelines. For instance, the European Union’s General Data Protection Regulation (GDPR) addresses the processing of personal data in AI applications. Additionally, various regulatory bodies, such as the Financial Stability Board and the International Organization of Securities Commissions, have issued reports highlighting the need for regulatory frameworks tailored to AI in finance.
Challenges of adapting regulations to AI
Adapting existing regulations to encompass AI in financial institutions poses several challenges. AI technologies evolve rapidly, often outpacing the development of regulatory frameworks. This leads to a regulatory lag, creating uncertainties for financial institutions and regulators alike. The complex, non-deterministic nature of AI algorithms also makes it challenging to enforce traditional rule-based regulations. Moreover, AI systems learn from vast amounts of data, sometimes from sources that may have inherent biases. Regulators must address these challenges to ensure that AI is used responsibly, ethically, and in compliance with relevant regulations.
Role of regulatory bodies and institutions in ensuring compliance
Regulatory bodies play a crucial role in overseeing the use of AI in financial institutions. To ensure compliance, regulators should collaborate with industry stakeholders to develop and implement appropriate regulatory frameworks. These frameworks should address the unique challenges posed by AI, while also considering existing regulations related to data protection, consumer rights, and fair lending practices. Regulatory bodies have the responsibility to monitor the use of AI in financial institutions, enforce compliance, and adapt regulations as technology evolves. By doing so, they can help foster public trust and ensure that AI is deployed ethically.
Ethical Concerns Associated with AI Implementation in Financial Institutions
Transparency and explainability of AI algorithms
One of the primary ethical concerns regarding AI implementation in financial institutions is the lack of transparency and explainability of AI algorithms. The complex, black-box nature of many AI models poses challenges in understanding how decisions are made. This opacity raises concerns about accountability, fairness, and potential biases. Financial institutions must prioritize the development and use of interpretable AI models and techniques to provide explanations for AI-driven decisions. Transparency in AI algorithms is essential to ensure that they can be understood, audited, and their decision-making processes can be verified.
Fairness and bias in decision-making processes
Ensuring fairness in decision-making is another crucial ethical concern in the use of AI in financial institutions. AI systems trained on historical data may perpetuate existing biases or produce discriminatory outcomes, leading to unfair treatment of certain individuals or groups. It is essential for financial institutions to identify and mitigate biases in training data and AI models. This can be achieved through rigorous testing, robust validation procedures, and ongoing monitoring of AI systems. Implementing fairness metrics and criteria can also help mitigate the risk of biased decision-making.
Data privacy and security in AI applications
The use of AI in financial institutions involves the processing and analysis of vast amounts of sensitive customer data. This raises significant ethical concerns regarding data privacy and security. Financial institutions must adhere to leading data privacy regulations and standards, such as the GDPR and relevant jurisdiction-specific data protection laws. They should implement robust data handling and storage practices, including encryption and access controls, to protect customer information from unauthorized access or data breaches. Data anonymization and de-identification techniques can further enhance privacy while allowing for data analysis and model training.
Accountability and responsibility in automated decision-making
AI systems in financial institutions often make automated decisions, ranging from credit approvals to investment recommendations. Ensuring accountability and responsibility in automated decision-making processes is a critical ethical concern. Financial institutions should define clear lines of responsibility and establish mechanisms for human oversight and review. Human operators should have the ability to intervene, verify decisions, and rectify any errors or biases. Legal frameworks must also be developed to address the potential liabilities and consequences of AI-driven decisions. Regular auditing and explaining automated decisions can enhance accountability and instill public trust.
Ensuring Fairness and Non-bias in AI Algorithms
Identifying and mitigating biases in training data
To ensure fairness and non-bias in AI algorithms, careful attention must be paid to the quality and representativeness of the training data. Financial institutions should thoroughly analyze training data to identify any biases present in the data samples. Biases can arise from historical patterns of discrimination or inadequate representation of certain groups. By identifying and addressing biases in the training data, institutions can mitigate the risks of biased outcomes. This includes employing diverse datasets and incorporating feedback loops to continuously measure and adjust for potential bias.
Implementing fairness metrics and criteria
To actively promote fairness in AI algorithms, financial institutions should establish clear fairness metrics and criteria. This involves defining objective measures and thresholds that determine whether an AI algorithm’s outcomes adhere to fairness standards. Financial institutions can leverage fairness metrics to monitor the performance of AI algorithms and identify any disparities or biases in decision-making. By regularly measuring and analyzing fairness metrics, institutions can proactively mitigate biases and strive for equitable and non-discriminatory outcomes.
Regular auditing and monitoring of AI systems
Continuous auditing and monitoring of AI systems are vital to ensuring fairness and non-bias. Financial institutions should develop robust auditing processes to evaluate the performance and behavior of AI algorithms. Through regular audits, institutions can identify and rectify biases, monitor compliance with fairness metrics, and ensure consistent adherence to ethical standards. Auditing also helps in identifying any unintended discriminatory impacts and provides opportunities for ongoing improvement and refinement of AI systems to minimize bias.
Addressing unintended discriminatory impacts of AI
Despite best efforts, AI systems can have unintended discriminatory impacts. Financial institutions must be proactive in addressing such impacts promptly and effectively. This includes actively monitoring AI systems for any biases that manifest in their decision-making processes. If unintended discriminatory impacts are identified, institutions should take immediate corrective action, such as modifying the algorithms, updating the training data, or engaging in additional human oversight. By addressing unintended biases promptly and transparently, financial institutions can foster trust and mitigate the potential harm caused by AI.
Transparency and Explainability in AI Systems
Interpretable AI models and techniques
To ensure transparency and explainability in AI systems, financial institutions should prioritize the use of interpretable AI models and techniques. Black-box models, such as deep neural networks, may produce accurate results but lack transparency. Interpretable AI models, on the other hand, provide explanations for their decision-making processes. Techniques such as rule-based models, decision trees, and model-agnostic interpretability methods can enhance the understandability of AI systems. By relying on interpretable models, financial institutions can provide explanations, audit AI-driven decisions, and address concerns around transparency and accountability.
Regulatory requirements for transparency and explainability
Regulators play a crucial role in defining and enforcing transparency and explainability requirements in AI systems. Financial institutions must comply with regulatory frameworks that mandate transparency in AI algorithms and decision-making processes. Regulators can require institutions to maintain records of AI models, data sources, and decision criteria, facilitating audits and ensuring accountability. By implementing regulatory requirements for transparency and explainability, financial institutions can build public trust and demonstrate a commitment to responsible AI use.
Using AI to enhance transparency in financial transactions
AI can also be leveraged to enhance transparency in financial transactions. Automated systems powered by AI can provide visibility into the process, ensuring that transaction details are recorded accurately and can be retrieved when needed. AI-powered systems can also analyze transaction patterns and detect anomalies, identifying potential risks or fraudulent activities. By utilizing AI to enhance transparency in financial transactions, institutions can contribute to a more secure and accountable financial ecosystem.
Ensuring understandable explanations for AI-driven decisions
Financial institutions must ensure that AI-driven decisions can be clearly explained to customers, regulators, and other stakeholders. Providing understandable explanations is crucial in instilling trust and mitigating concerns around the black-box nature of AI algorithms. Institutions can develop user-friendly interfaces that present explanations in a transparent and accessible manner. Additionally, institutions should invest in educating their customers and stakeholders about AI systems, their limitations, and the decision-making processes involved. By focusing on clear and understandable explanations, financial institutions can address transparency concerns and promote ethical AI practices.
Data Privacy and Security Concerns in AI
Leading data privacy regulations and standards
Data privacy regulations play a critical role in ensuring the ethical use of AI in financial institutions. Financial institutions must adhere to leading data privacy regulations and standards, such as the GDPR, which provide guidelines for the collection, processing, and storage of personal data. These regulations require institutions to obtain consent, implement data protection measures, and notify individuals about how their data is being used. By complying with data privacy regulations, financial institutions can protect customer privacy while harnessing the benefits of AI.
Protecting sensitive customer information in AI systems
The use of AI in financial institutions involves the processing of vast amounts of sensitive customer information, including financial transactions, personal details, and credit history. Financial institutions must prioritize the protection of this information. Robust security measures, such as encryption, role-based access controls, and secure data handling practices, should be implemented to prevent unauthorized access or data breaches. Institutions should also ensure secure data transmission and storage to maintain the confidentiality and integrity of customer information throughout the AI system’s lifecycle.
Secure data handling and storage practices
Financial institutions must establish secure data handling and storage practices to safeguard customer information. The classification and categorization of data based on its sensitivity enable institutions to adopt appropriate security measures. Encryption algorithms can be used to protect data both in transit and at rest. Access controls and authentication mechanisms should be implemented to restrict access to authorized personnel only. Regular security assessments and penetration testing can help identify vulnerabilities and ensure continuous improvement in data handling and storage practices.
Data anonymization and de-identification techniques
To further protect customer privacy, financial institutions can employ data anonymization and de-identification techniques. These techniques involve removing or modifying personally identifiable information from datasets, rendering them less susceptible to identification. By anonymizing data, financial institutions can retain the utility of data for AI applications while minimizing the risk of re-identification and maintaining privacy. However, it is important to ensure that anonymization techniques are properly implemented, as re-identification attacks can still occur. Balancing privacy and utility is instrumental in addressing data privacy concerns in AI applications.
Accountability and Responsibility in Automated Decision-Making
Defining responsibility in AI systems
Defining responsibility in AI systems is crucial to ensure accountability and ethical practices. Financial institutions should clearly delineate the roles and responsibilities of both human operators and the AI system itself. While AI systems can automate decision-making processes, human operators are responsible for overseeing and validating AI-driven decisions. Institutions should establish guidelines and frameworks to clearly communicate this division of responsibility, providing clarity on the extent to which AI systems are accountable for their decisions.
Establishing clear lines of accountability
Clear lines of accountability between financial institutions and AI systems are necessary to address ethical concerns. Institutions should establish mechanisms to monitor and assess AI system performance, ensuring alignment with ethical guidelines and regulatory requirements. Accountability should be shared between human operators, who oversee and interpret AI-driven decisions, and the AI system itself. By defining and enforcing clear lines of accountability, financial institutions can ensure that AI is used responsibly and that decision-making processes can be audited and explained.
Legal implications of AI decisions
AI decisions in financial institutions can have legal implications, raising important ethical considerations. Institutions must ensure that AI systems comply with applicable laws and regulations, including those related to anti-discrimination, consumer protection, and fair lending practices. It is crucial to establish legal frameworks that address potential liabilities arising from AI-driven decisions. Institutions should collaborate with legal experts to identify and mitigate potential legal risks associated with AI use in finance, ensuring that AI decisions align with legal norms and ethical standards.
Developing frameworks for auditing and explaining automated decisions
To enhance accountability and transparency, financial institutions should develop frameworks for auditing and explaining automated decisions. This involves establishing processes to review AI-driven decisions, assess their fairness and compliance, and identify potential biases. Audit logs, documentation, and data recording should be maintained to facilitate transparency, accountability, and regulatory compliance. Institutions should explore explainability techniques to provide understandable and verifiable explanations for AI-driven decisions. By implementing frameworks for auditing and explaining automated decisions, financial institutions can ensure compliance, foster trust, and address ethical concerns.
Risk Management and Compliance Strategies for AI in Financial Institutions
Risk assessment and mitigation in AI applications
Financial institutions must conduct thorough risk assessments to identify and mitigate potential risks associated with AI applications. This entails evaluating the potential impact of AI-driven decisions on customers, employees, and the institution itself. Institutions should establish risk management frameworks that include comprehensive identification, assessment, and mitigation strategies specific to AI. This involves assessing the accuracy, reliability, and potential biases of AI algorithms, as well as the potential operational, reputational, and regulatory risks associated with their use. Robust risk management practices are crucial to ensure ethical deployment of AI in financial institutions.
Compliance frameworks and best practices
To ensure compliance with regulatory requirements and ethical standards, financial institutions should develop comprehensive compliance frameworks tailored to AI applications. Compliance frameworks should incorporate guidelines for transparency, fairness, data privacy, security, and accountability. Leveraging best practices from industry leaders and regulatory guidance, institutions can enhance their compliance strategies. It is important to regularly review and update compliance frameworks to keep pace with evolving regulatory landscape and technological advancements. By incorporating compliance frameworks and best practices, financial institutions can mitigate risks and demonstrate their commitment to ethical practices.
Monitoring and auditing AI systems for compliance
Continuous monitoring and auditing of AI systems are essential to ensure adherence to ethical practices and regulatory requirements. Financial institutions should establish robust monitoring mechanisms to detect and address potential violations or deviations from ethical standards. Regular audits should be conducted to assess the performance, behavior, and compliance of AI algorithms. Compliance teams should collaborate closely with AI teams to ensure that monitoring processes are seamlessly integrated. By proactively monitoring and auditing AI systems, financial institutions can mitigate compliance risks and ensure ethical AI practices.
Collaboration between compliance and AI teams
Collaboration between compliance and AI teams is vital to ensure effective risk management and adherence to ethical standards. Compliance teams possess the knowledge and expertise in regulatory requirements, while AI teams are responsible for developing and deploying AI systems. By fostering collaboration and communication between these teams, financial institutions can ensure that compliance concerns are addressed during the development and implementation of AI applications. Compliance experts can provide guidance on data privacy, transparency, fairness, and other ethical considerations, enabling the AI teams to incorporate these principles into their systems.
Human Oversight and Regulatory Challenges
Balancing automation and human intervention
Achieving the right balance between automation and human intervention is a regulatory challenge in the use of AI in financial institutions. While automation can enhance efficiency and accuracy, human oversight is necessary to address ethical concerns, ensure compliance, and provide accountability. Regulatory bodies must determine the appropriate level of human intervention required in critical decisions and establish guidelines that strike a balance between efficient automation and necessary human oversight. Striking this balance depends on the nature of the financial process, potential risks involved, and the impact on customers and stakeholders.
Regulatory requirements for human oversight
Regulatory requirements for human oversight in AI systems vary across jurisdictions and financial sectors, reflecting the ongoing regulatory challenges. Some jurisdictions mandate human involvement in sensitive financial decisions, such as loan approvals or investment recommendations. Regulatory bodies must carefully consider the extent of human oversight required, acknowledging the limitations and capabilities of AI systems. By developing regulatory requirements for human oversight, regulators can address ethical concerns and ensure that AI is used responsibly and in compliance with applicable laws.
Responsibilities of human operators in AI systems
Human operators play a critical role in ensuring ethical practices and compliance in AI systems. In financial institutions, human operators are responsible for overseeing AI-driven decision-making processes and validating the outcomes. Human operators should be trained to understand the limitations and potential biases of AI algorithms, be equipped with the necessary tools to interpret and explain AI-driven decisions, and possess the authority to intervene when necessary. By clearly defining and communicating the responsibilities of human operators, financial institutions can cultivate a culture of responsible and accountable AI use.
Addressing challenges of relying solely on AI
Relying solely on AI systems poses ethical challenges and regulatory concerns in financial institutions. The black-box nature of AI algorithms and the potential for biases and errors necessitate human oversight. Institutions must recognize the limitations of AI and the need for human judgment, intuition, and ethical decision-making. By acknowledging the challenges of relying solely on AI and ensuring the appropriate level of human intervention, financial institutions can strike a balance between technological advancement and responsible use of AI.
Collaboration between Financial Institutions and Regulators
Engagement and cooperation with regulatory authorities
Financial institutions should actively engage and cooperate with regulatory authorities to address the challenges associated with AI compliance and regulation. This involves participating in public consultations, sharing insights, and providing feedback on proposed regulations related to AI. Collaboration between financial institutions and regulators can facilitate a deeper understanding of the risks and benefits of AI in finance. By actively engaging with regulatory authorities, financial institutions can shape regulatory frameworks that foster innovation, ensure compliance, and uphold ethical practices.
Shaping regulatory frameworks through industry involvement
Financial institutions have a responsibility to shape regulatory frameworks through industry involvement and collaboration. Institutions should proactively contribute to industry associations, working groups, and standard-setting bodies to share best practices, lessons learned, and insights on AI compliance. By working collectively, financial institutions can influence the development of regulatory frameworks, ensuring that they are fair, balanced, and tailored to address the unique challenges posed by AI in finance. Collaboration among industry stakeholders is instrumental in shaping regulatory frameworks that promote ethical practices and enable responsible AI use.
Sharing best practices and lessons learned
Financial institutions should actively share best practices and lessons learned to foster a culture of continuous improvement and knowledge sharing. As AI technologies evolve, financial institutions encounter various challenges, successes, and innovative approaches. Sharing experiences and insights can help other institutions navigate these challenges and adopt ethical practices. By sharing best practices, financial institutions can collectively contribute to the development of industry-wide standards and guidelines, promoting responsible and compliant AI adoption.
Establishing channels for continuous dialogue
Open channels of communication and continuous dialogue between financial institutions and regulatory authorities are essential to ensure effective regulation and compliance. Regular meetings, forums, and consultations provide opportunities for institutions to seek clarifications, raise concerns, and provide feedback on regulatory initiatives. Establishing mechanisms for ongoing dialogue fosters collaboration, improves mutual understanding, and enables regulators to keep pace with technological advancements. Continuous dialogue facilitates the development of regulatory frameworks that address emerging challenges and uphold ethical practices in AI adoption.
In conclusion, AI in financial institutions offers immense potential for streamlining processes, enhancing decision-making, and improving customer experiences. However, ensuring ethical practices in AI implementation requires addressing transparency, fairness, data privacy, security, accountability, and regulatory compliance. Financial institutions must collaborate with regulatory bodies, invest in interpretable AI models, identify and mitigate biases, establish robust risk management and compliance strategies, and strike a balance between automation and human oversight. Through proactive ethical considerations and adherence to regulatory frameworks, financial institutions can harness the benefits of AI while mitigating risks and establishing public trust in the financial industry’s AI-driven future.